Doing understanding differently: Rethinking our relationship with metrics – and the people behind the data
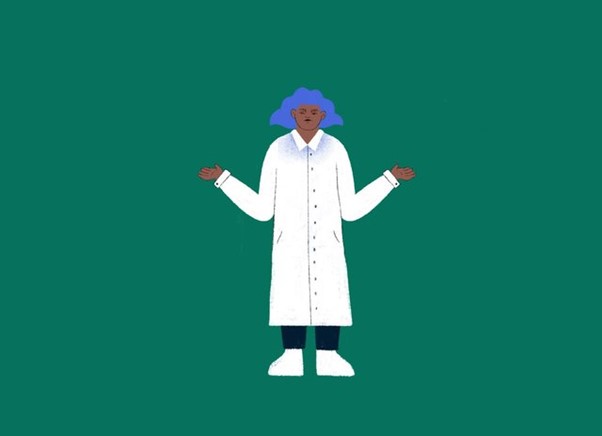
This article was first commissioned and published by The Sociological Review
“We are looking for the gold standard metric of social mobility.”
This was one of the opening lines in the introductory meeting for my postdoctoral fellowship, which was in partnership with a non-departmental body. It was an intimidating proposition for a recent PhD student in the critical sociology of metrics and policy. Was I the right person to find it for them? Did I even understand what they meant?
I have become more persuasive in advocating for doing metrics differently: better clarification, communication and consideration is needed.
Curious, I Googled the phrase “gold standard metric”. According to the first result, “a gold standard study may refer to an experimental model that has been thoroughly tested and has a reputation in the field as a reliable method”.
So, not even a metric, but a study, and it may mean numerous things, but it has status as reliable. Or, as science historian Theodore Porter would say, a number we can put trust in.
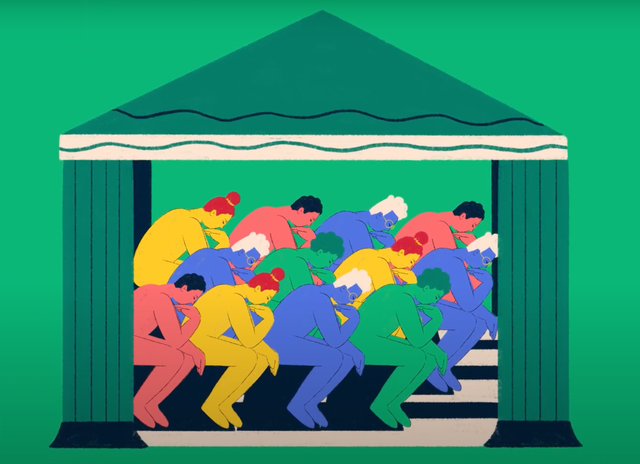
From the animated series ‘Understanding Well-being Data’, written by Susan Oman, graphics Carmela Caldart, animations, Kieran Bentley, produced by Sean Lovell.
Crucially, then, a gold standard metric is contingent on the field – and this tentative definition suggests there may not be a universal understanding of its definition at all. I wasn’t aware back in the meeting – before the fieldwork, the process of distilling the findings and my reflections when writing a monograph – but there is much to consider about the relationship between metrics and understanding.
Some people might be happy to collect data, and then analyse it to derive understanding that will be shared through metrics. The limitations of this approach and ways in which metrication compromises certain forms of knowledge have been long documented.
My data science students often arrive having learned something called a DIKW pyramid. This visual representation of a framework describes a linear process starting with “Data” (the D), from which we gather “Information” that leads to “Knowledge”, implying that the W for “Wisdom” is dependent on this linear process. Together we reflect on the limitations of these positivist linear journeys and I encourage them to think of, and understand, metrics differently.
Moments of miscommunication
Returning to my online search for the gold standard metric of social mobility: words like “experimental” and “thoroughly tested” stood out. If I was going to understand how any metric of social mobility would work for those being measured, I would need to test different questions used to understand social mobility – and thoroughly, with real people – and see how that compared to the literature review of the measurers. How might they understand the questions and metrics differently?
When we talk of the effects of datafication (the increasing use of data), we often fixate on how metrics classify people in a way that assumes they might only attain certain grades at school, for example. We also advocate against the impact these processes have on access to welfare or healthcare. Yet we rarely consider how people experience data collection; it can be a crucial moment of misrepresentation or miscommunication. As this affects both participants and the data, reflecting on this process is critical for understanding.
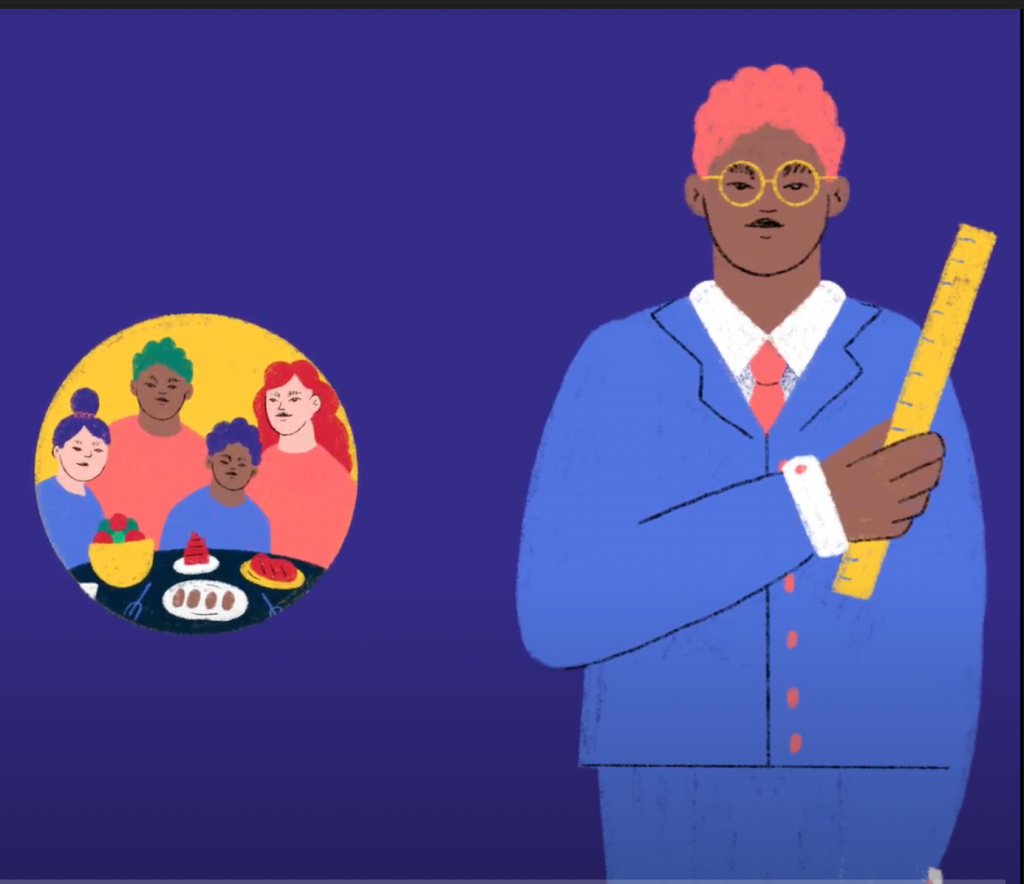
From the animated series ‘Understanding Well-being Data’, written by Susan Oman, graphics Carmela Caldart, animations, Kieran Bentley, produced by Sean Lovell.
With social mobility metrics, this often means asking people about their social origins. These questions will often be presented alongside other, more familiar questions pertaining to race, gender, sexuality, disability, faith and age. By trialling a series of questions used to collect proxy data for class and social mobility, embedded in simulated equality monitoring questionnaires, I was able to “test” them in various ways.
I used this technique in group discussions with 126 co-workers in the cultural sector where the metric was to be introduced, to find out how people experience equality monitoring in these workplaces. I also did one-to-one interviews with people who processed these data, and those responsible for them at strategic level. This layered approach to understanding the metrics revealed different understandings of the value of these data, what they were for and how personal they felt to the interview subjects.
Despite being about understanding, this was not a theoretical exercise, but an experiential, relational and empirical one. It was informed by listening to how others come to understand and how they experience metrics, while also observing the processes and practices of metrication and their ways of seeing. Yet it is important to reflect on something often overlooked in research: the word “understanding” has many meanings that we don’t often consider. It can mean:
- the ability to understand something; comprehension
- abstract thought and intellect
- an individual’s perception or judgement of a situation: “Well, I understood otherwise”
- indicate sympathetic awareness: “They did it, and with understanding for others”
- an informal or unspoken agreement: a shared understanding
- in its ancient meaning, to have insight or good judgement
When it comes to metrics, we often consider the first meanings – ability to understand something, comprehension and abstract intellect – as lying in the skills and training of social or data scientists. Crucially, we often don’t think of the need for survey designers or analysts to understand the experience of equality monitoring processes. Yet as one participant argued: “I find these kinds of forms uncomfortable. Less to do with me but more to do with I know that they’re seeking out information about people.”
This discomfort is not personal, it’s not about their personal circumstances, but derives from their understanding that the question is there to seek out personal information. Their perception of the relationship between questions, data and information complicates that linear DIKW pyramid model. Contrast this with some of my data science students’ knowledge of that framework, generated to inform a shared understanding across the field.
Questions as proxies
What of the shared understanding of social scientists, with their qualifications of what deserves the “reputation” for having a “reliable method” of measuring social mobility? In the case of the question long used in the UK’s Office for National Statistics surveys, this was inherited from sociologist John Goldthorpe’s work. This approach to understanding social mobility and class uses the following question to generate what is understood to be the most robust metric: what was the occupation of your main household earner when you were about age 14?
This question provoked the most discussion, and each group took issue with it. They imagined what metrics were trying to do, demonstrating their independent route to achieving understanding, alongside posing many questions. They asked a variation of the following: “What are you trying to get at?” “Why my parents, not me?” “Why 14?” “Why the employment of only one?” “What if I don’t know the main earner?” “What about the information that this question does not capture?”
They seemed instinctively to grasp the proxy nature of the question (although they did not use the word “proxy” themselves), and to understand that it wasn’t these details themselves that were of value, but the fact that they would somehow help someone else understand something. Crucially, the rationale and value were not shared, which led discussants to question the practical limitations of the questions and the metrics.
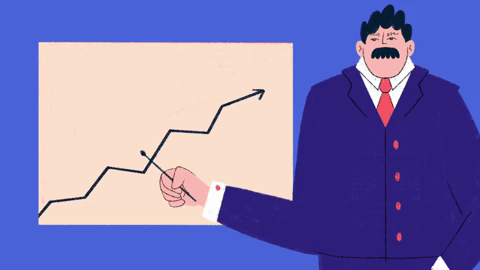
From the animated series ‘Understanding Well-being Data’, written by Susan Oman, graphics Carmela Caldart, animations, Kieran Bentley, produced by Sean Lovell.
As well as imagining how the metrics work and sharing their practical concerns, people also considered the processes of questioning personally, as above – and on behalf of others. People imagined potential harms and asked: “What if people don’t want to remember the age 14?” “What if something bad happened to them at that age?” “What if their parents kicked them out?” Others made the more explicit political point that it was unethical to ask these questions.
“This is a project of care, it’s about trying to make the sector a better place for everyone, but somehow the way it is done is the opposite. It’s unfriendly and, I think, can feel hostile,” said one interviewee, a director of a national museum. She supported the attempt to understand inequality in order to achieve positive social change, but recognised how the approach lacks understanding for others.
Feeling for others
Some, when thinking about the processes of metrication, do so with understanding of others – their empathy causes them to imagine the experience of these processes for people who might be harmed. By contrast the survey designers, and those who use metrics, whether researchers or policy-makers, often lack understanding of the origins of the data that make the metrics: us.
We rarely consider how people experience data collection; it can be a crucial moment of misrepresentation or miscommunication.
Writing up the metrics project for different audiences, through presentations, policy briefings, working papers, journal articles, animated films and an open access book has changed the way I understand the concept of understanding in relation to metrics. I have become more persuasive in advocating for doing metrics differently: better clarification, communication and consideration is needed, as is attending to people’s practical, personal and political issues with the processes.
For that reason, rethinking understanding in metrication contexts can be critical and reparatory. It can allow us to reach that final meaning of understanding: having insight or good judgement on how to address social inequality.
References and further reading
- Back, L. (2007). The Art of Listening. Bloomsbury.
- Cardoso, J. R., Pereira, L. M., Iversen, M. D. & Ramos, A. L. (2014). What is gold standard and what is ground truth?. Dental Press J Orthod, 19(5): 27–30. http://doi.org/10.1590/2176-9451.19.5.027-030.ebo
- Oman, S. (2021). Understanding Well-being Data: Improving Social and Cultural Policy, Practice and Research. Palgrave Macmillan Cham.
- Oman, S. (2022). Re-performance: a critical and reparative methodology for everyday expertise and data practice in policy knowledge. International Review of Public Policy. https://doi.org/10.4000/irpp.1833
- Porter, T. (1996) Trust in Numbers. Princeton University Press
- Savage, M., & Burrows, R. (2007). The Coming Crisis of Empirical Sociology. Sociology, 41(5), 885–899. https://doi.org/10.1177/0038038507080443
- Scott, J. (1999) Seeing like a State: How Certain Schemes to Improve the Human Condition Have Failed. Yale University Press.
About the author
Susan Oman is Lecturer in Data, AI and Society at the University of Sheffield, and co-investigator on the project Living With Data. She researches how data and evidence work – for and against different people – in context and in practice, looking at policy issues such as wellbeing and inequality. Dr Oman focuses on making her work accessible to different audiences, and her open-access monograph Understanding Well-being Data: Improving Social and Cultural Policy, Practice and Research (2021) has been translated into animations, podcasts and a website at well-beingdata.com. Twitter: @Suoman
Cite this work
Oman, S. (2022, July 5). Doing understanding differently: Rethinking our relationship with metrics – and the people behind the data [Online]. The Sociological Review Magazine. https://doi.org/10.51428/tsr.ndhx3631
Copyright
© 2022 Susan Oman. This work is licensed under The Sociological Review Free Access Licence.